Keynote Speakers of MICAD 2024
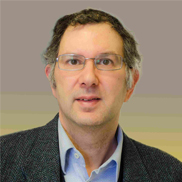
Prof. Laurent Cohen
IEEE Fellow
Universite Paris Dauphine, France
Laurent David Cohen was born in 1962. He was student at the Ecole Normale Superieure , rue d'Ulm in Paris, France from 1981 to 1985. He received the Master's and Ph.D. degrees in Applied Mathematics from University of Paris 6, France, in 1983 and 1986, respectively. He got the Habilitation à diriger des Recherches from University Paris 9 Dauphine in 1995.
He obtained in 1990 a position of Research Scholar (Charge then Directeur de Recherche 1st class) with the French National Center for Scientific Research (CNRS) in the Applied Mathematics and Image Processing group at CEREMADE, Universite Paris Dauphine, Paris, France. His research interests and teaching at university are applications of Partial Differential Equations and variational methods to Image Processing and Computer Vision, like deformable models, minimal paths, geodesic curves, surface reconstruction, Image segmentation, registration and restoration.
For many years, he has been editorial member of the Journal of Mathematical Imaging and Vision, Medical Image Analysis and Machine Vision and Applications.
He was also member of the program committee for about 50 international conferences. He authored about 300 publications in international Journals and conferences or book chapters, and 6 patents.
In 2002, he got the CS 2002 prize for Signal and Image Processing. In 2006, he got the Taylor & Francis Prize:
"2006 prize for Outstanding innovation in computer methods in biomechanics & biomedical engineering."
He was 2009 laureate of Grand Prix EADS de l'Academie des Sciences
He was promoted IEEE Fellow 2010 for contributions to computer vision technology for medical imaging
Speech title: Fast marching and front propagation for medical image segmentation
Abstract: Minimal paths have been used for long as an interactive tool to find contours or segment tubular and tree structures, like vessels in medical images. The user usually provides start and end points on the image and gets the minimal path as output, as a cost minimizing curve. These minimal paths correspond to minimal geodesics according to some relevant metric defined on the image domain. Finding geodesic distance and geodesic paths can be solved by the Eikonal equation using the fast and efficient Fast Marching method.
Minimal paths are a way to find the global minimum of a simplified active contour energy. In the past years, we have extended the minimal path methods with asymmetric Randers Metrics to cover all kinds of active contour energy terms, as well as segmentation by front propagation. For example, a way to penalize the curvature in the framework of geodesic minimal paths was introduced, leading to more natural results in vessel extraction.
Recently, we introduced new methods combining the efficiency of minimal paths with CNN. In a first method, CNNs are trained to find a set of keypoints and minimal paths are found that link these keypoints. In another context, CNNs are used to generate relevant metrics adapted to a problem. We will present various applications to medical image segmentation.
Prof. Gustavo Carneiro
University of Surrey, UK
Professor of AI and Machine Learning
Centre for Vision, Speech and Signal Processing (CVSSP)
Gustavo Carneiro is a Professor of AI and Machine Learning at the University of Surrey, UK. Before that, from 2019 to 2022, he was a Professor at the School of Computer Science at the University of Adelaide, an ARC Future Fellow, and the Director of Medical Machine Learning at the Australian Institute of Machine Learning. He joined the University of Adelaide as a senior lecturer in 2011, has become an associate professor in 2015 and a professor in 2019. In 2014 and 2019, he joined the Technical University of Munich as a visiting professor and a Humboldt fellow. From 2008 to 2011 Dr. Carneiro was a Marie Curie IIF fellow and a visiting assistant professor at the Instituto Superior Tecnico (Lisbon, Portugal) within the Carnegie Mellon University-Portugal program (CMU-Portugal). From 2006 to 2008, Dr. Carneiro was a research scientist at Siemens Corporate Research in Princeton, USA. In 2005, he was a post-doctoral fellow at the University of British Columbia and at the University of California San Diego. Dr. Carneiro received his Ph.D. in computer science from the University of Toronto in 2004. Prof. Gustavo Carneiro has a wide-range of research interests. He is interested in applied research in medical image analysis and computer vision to theoretical research in machine learning.
Speech Title: People-Centred Medical Image Analysis
Abstract: Medical image classification is a complex task, where even expert annotators can sometimes be uncertain about the classes present in the images. This uncertainty introduces the challenge of learning with noisy labels (LNL). Addressing LNL is inherently difficult, as it requires either making strong assumptions or incorporating multiple noisy labels for each training image. While these approaches can produce models that perform well in controlled settings, they often fall short when compared to consensus-driven human classification. A promising alternative is human-AI collaborative classification (HAI-CC), which seeks to explore the complementary strengths of human expertise and AI. However, the intricate decision-making required to fully exploit these synergies makes HAI-CC a challenging task. HAI-CC typically involves three possible modes of interaction: 1) AI classifies independently, 2) learning to complement, where AI collaborates with users, and 3) learning to defer, where AI defers to human judgment. Despite their interrelated nature, these modes have often been studied in isolation rather than as part of an integrated system. Furthermore, a key limitation in the "learning to complement" mode is that AI models tend to oversimplify the problem by using a global human behaviour model, ignoring individual variability. This leads to suboptimal performance. In this talk, we will discuss recent advancements from our lab in HAI-CC, particularly a system that unifies the three modes of HAI-CC and another system that introduces a "learning to complement" approach that adapts to individual human behaviour models, improving collaboration and decision-making.
Prof. James C. Gee
University of Pennsylvania, USA
Director, Penn Image Computing and Science Laboratory
Prof. Gee is the Director of Penn Image Computing and Science Laboratory, Department of Radiology, Perelman School of Medicine, University of Pennsylvania, Philadelphia, PA. He is the Co-Director of the Translational Biomedical Imaging Center at the Institute of Translational Medicine and Therapeutics at the University of Pennsylvania. He is also the Director of the Interfaces Program in Biomedical Imaging and Informational Sciences at UPenn. Dr. Gee's major area of interest is biomedical image analysis and computing, with active research in all of the quantitative methods represented, including segmentation, registration, morphometry and shape statistics, as applied to a variety of organ systems and all of the major and emerging modalities in biological/biomaterials imaging and in vivo medical imaging.
Speech Title: Registration-aware feature learning
Prof. Xiaoyi Jiang
University of Münster, Germany
Head of Group
Xiaoyi Jiang studied Computer Science at Peking University, China, and received his PhD and Venia Docendi (Habilitation) degree from University of Bern, Switzerland. He was associate professor at Technical University of Berlin and since 2002 full Professor at University of Münster, Germany. Currently, he is the dean of Faculty of Mathematics and Computer Science at University of Münster. He is a PI and research area leader of the Cluster of Excellence “Cells in Motion – Imaging to understand cellular behaviour in organisms” established by the German Research Foundation DFG in 2013. He is Editor-in-Chief of International Journal of Pattern Recognition and Artificial Intelligence. In addition, he also serves on the Advisory Board and Editorial Board of several journals, including IEEE Transactions on Medical Imaging, International Journal of Neural Systems, Pattern Analysis and Applications, and Pattern Recognition. His research interests include biomedical imaging, 3D image analysis, and structural pattern recognition. He is a Senior Member of IEEE and Fellow of IAPR.
Speech Title: Traditional computer vision meets deep learning: A perspective on biomedical image analysis
Abstract: Deep learning has revolutionized computer vision, significantly advancing the field of biomedical image analysis, where it now plays a dominant role. However, traditional computer vision techniques, developed over decades, still hold valuable potential. While these methods may not always match the performance of deep learning, they offer distinct advantages, such as reduced data collection efforts, lower computational requirements, and improved explainability. More importantly, combining traditional approaches with deep learning can result in mutual benefits. This talk will explore synergistic strategies for integrating these techniques, with a focus on biomedical image segmentation. Main traditional methods under discussion include dynamic programming, active shape/appearance models, active contours, and level sets.